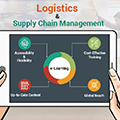
The educational landscape has experienced significant evolution over the past decade, with one of the most profound changes being the integration of technology into learning environments. Among the many technological advancements, Machine Learning (ML) stands out as a pivotal force driving innovation in education technology (EdTech). From personalized learning experiences to predictive analytics, ML is reshaping how students learn and educators teach.
Machine Learning, a subset of artificial intelligence (AI), involves the development of algorithms that enable computers to learn from and make decisions based on data. Unlike traditional programming, where explicit instructions are given to solve a problem, ML algorithms learn from examples, identifying patterns and making predictions based on that data.
In the context of EdTech, ML is employed to analyse vast amounts of educational data, tailoring learning experiences to individual students, optimizing administrative tasks, and even predicting future learning outcomes. This ability to adapt and evolve based on user interaction makes ML an invaluable tool for modern education.
One of the most significant contributions of ML to EdTech is the creation of personalized learning experiences. Traditional education often follows a one-size-fits-all approach, which can leave some students behind while others become disengaged due to lack of challenge. ML addresses this issue by analysing data on a student's learning style, pace, strengths, and weaknesses. It can then recommend resources, adjust difficulty levels, and even create customized learning paths that cater to the unique needs of each student.
For example, platforms like Dream Box and Knew ton use ML algorithms to assess a student's performance in real-time and adapt the content accordingly. This ensures that students remain engaged, receive the appropriate level of challenge, and are supported in areas where they struggle.
Intelligent Tutoring Systems (ITS) represent another significant application of ML in EdTech. These systems provide students with one-on-one tutoring by simulating the guidance of a human tutor. ML algorithms allow ITS to assess a student’s knowledge, provide instant feedback, and offer hints or additional resources as needed.
Carnegie Learning’s Mathia, for instance, is an ITS that uses ML to deliver personalized math instruction. It continuously analyses a student's progress and adapts the learning experience to ensure mastery of concepts, mimicking the responsiveness of a human tutor.
Predictive analytics is a powerful ML application in education, helping educators and institutions identify at-risk students and intervene before problems escalate. By analysing historical data, including grades, attendance, and even social factors, ML models can predict which students are likely to struggle or drop out. This enables proactive measures, such as targeted support or counselling, to be implemented early on.
Institutions like Georgia State University have successfully used predictive analytics to increase retention and graduation rates. By identifying students who might be at risk of failing or dropping out, the university can provide the necessary support to keep them on track.
The grading process is often time-consuming and subject to human bias. ML offers a solution through automated grading systems that can assess student work, particularly in subjects like mathematics and science, where answers are more standardized. For more subjective areas like essay writing, ML algorithms can evaluate structure, grammar, and even the relevance of content, providing instant feedback to students.
Platforms like Grade scope use ML to streamline the grading process, saving educators time and ensuring more consistent and objective assessments. This also allows students to receive timely feedback, which is crucial for their learning progression.
ML is also playing a critical role in making education more accessible and inclusive. Speech recognition, text-to-speech, and translation algorithms powered by ML are helping to break down language barriers and support students with disabilities. For instance, students with visual impairments can use screen readers enhanced by ML to access educational content, while those with hearing impairments can benefit from real-time captioning powered by speech recognition algorithms.
Moreover, ML-driven platforms can offer content in multiple languages, catering to a diverse student population and ensuring that everyone has equal access to quality education.
While the benefits of ML in EdTech are substantial, they are not without challenges. Issues such as data privacy, algorithmic bias, and the digital divide must be addressed to ensure that ML-enhanced educational tools are used responsibly. Additionally, educators must be adequately trained to effectively integrate these technologies into their teaching practices.
Ethical considerations are paramount, particularly concerning how data is collected, stored, and used. Transparency in algorithmic decision-making and efforts to minimize bias in ML models are essential to building trust among educators, students, and parents.
As ML continues to evolve, its impact on education will only grow. Future advancements may include even more sophisticated adaptive learning systems, virtual reality (VR) experiences tailored by ML algorithms, and deeper integration of AI tutors in everyday classroom settings. The goal will always be to enhance the learning experience, making it more engaging, personalized, and effective.
Machine Learning is revolutionizing EdTech by enabling personalized learning, improving student outcomes through predictive analytics, and streamlining administrative tasks. As we navigate the challenges and opportunities of this technology, it is clear that ML will be central to the future of education, driving innovation and making learning more accessible and effective for all.
The integration of Machine Learning into EdTech is transforming the educational landscape, offering unprecedented opportunities for personalized learning, enhanced student support, and greater accessibility. As ML continues to advance, it holds the potential to make education more effective, engaging, and inclusive, catering to the diverse needs of students worldwide. However, as we embrace these innovations, it is crucial to address the ethical and practical challenges that accompany them, ensuring that the benefits of ML in education are realized responsibly and equitably. The future of education is undoubtedly intertwined with the evolution of Machine Learning, promising a more tailored and dynamic learning experience for generations to come.
Neti is a dynamic professional with a diverse background in human resources, operations, and personality development training. Recognized as a transformative leader, she empowers others as a sought-after mentor, leveraging her deep understanding of human behavior. An avid reader and traveler, she applies her global mindset to innovative problem-solving in the e-learning industry, where she leads the development of cutting-edge educational platforms. Her journey exemplifies versatility, resilience, and a commitment to personal and professional growth.
Leave a comments